Tryst with Data
- Kriti Bajpai
- Feb 27
- 8 min read
Rule four : Consider everything an experiment.
I have been a writer for as long as I’ve had thoughts I couldn’t say out loud. Maybe even before that. Writing feels less like a choice and more like a condition—like nearsightedness or an allergy that needs some scratching.
We were asked for a detailed description of our experience so far. I assume they meant something useful, not, “It all began in the third grade when I wrote a poem about a tree.” And so, instead, to begin with, I will tell you this: I write because I must. Because long ago, someone did, and the world became larger for it. I write because the alternative is unbearable. I write because language is the only leash I have on reality, and even then, it slips. I write because when I don’t, I feel like a badly drawn character in someone else’s story.
The GE GenAI Chatbot project was uncharted territory. I had never written for a chatbot—let alone as one. But if there’s one thing I learned in the year-long process of co-building a sentient bot, it’s this: to create one, you have to become one. Which, in essence, means you take your own emotions, break them down into something almost clinical, almost mechanical, and feed them into the system. Pain, joy, confusion—all turned into data points. And then, somehow, that data breathes. I have to thank Shruti for that. They put me in touch and had more confidence in me than I did at the time—or at least more than I could grasp. That's a lot of grace. We’re also flatmates now. Thank you, Shroom-tea.
Here, let me quickly explain the project.
Girl Effect owns three chatbots as part of its IP: BigSis for South Africa, Wazzii for Kenya, and BolBehen for India. The first chatbot we worked on was BigSis—a digital guide designed to provide crucial, reliable information on mental, sexual, and reproductive health.
BigSis isn’t just a chatbot. It’s a carefully designed companion, built to address both everyday and deeply sensitive topics—friendships, relationships, sex, abortion, menstruation, abuse, assault, threats, and denied health access. In a world that often suppresses self-expression, BigSis fosters understanding. In an age of misinformation, it offers a safe, reliable space for young girls and boys who are often neglected.
The project started with data. A lot of data. When Soma and I first spoke, one of my initial questions was, “How much data are we looking at?” Soma, ever pragmatic, had no definitive answer. But Soma is honest. The truth was, no one really knew. It was uncharted territory for everyone. And that, in some strange, exhilarating way, made it all the more thrilling.
Oh, let’s not forget the designation negotiation.
I was hired as a content writer. Then, like all things in tech, the role shape-shifted. Content writer became UX and content writer. Then, as the work stacked up and the job descriptions blurred, I became a data curator. And finally—after enough time measured not in months, but in sheer volumes of data—we found ourselves here.
Data annotators. Project GenAI. The architects of meaning in a machine that was learning to think.
They should just call us mothers. What is training a chatbot if not mothering a newborn? You teach it language, boundaries, a sense of the world. You correct its mistakes, watch it fumble, hope it doesn’t embarrass you in public.
On paper, though, we’re still UX and Content Writers. Well.
To be fair, one of the briefs around the foundation of the bot was to make it sound like an elder sister with whom you can share concerns without fearing judgement. Hence the name–BigSis.
No project is a solo journey. Behind every trained chatbot—every faceless child of AI—there’s a team with names, hearts, and an unhealthy attachment to spreadsheets. In this case, that team was Soma Mitra Behura, Amalia Villa, Liz Otieno, and me, Kriti Bajpai.
Liz, from Kenya, shared my role, and we became a solid team. She’s sharp, perceptive, and absurdly funny—the kind of funny that belongs to a 90-year-old war-surviving grandma who’s seen it all and decided to laugh at it.
Our first task? Data cleaning. Sounds simple, right? Read, assess, edit, delete, collate. Except this data had layers—messy, unnecessary, tangled. We hacked away at it through multiple iterations, which essentially meant an ever-growing graveyard of Excel sheets named 1236489_FINAL_FINAL.
As they say, all great things start on an Excel sheet. Who says that? I didn’t. Did you? Well, somebody. Or maybe I just made it up.
Anyway,
The second step—safeguarding.
When your data involves sensitive, high-stakes topics, safeguarding isn’t just a step—it’s the step. We were handed multiple datasets: trigger words, risk levels, safeguarding sentences, vetted content, uncaught messages. Our task? To craft 60 new questions, split into three categories:
20 sensitive disclosure questions
20 non-sensitive questions
20 random questions
Emotionally? Triggering. Cognitively? Demanding. Methodologically? Precise, because every single question carried weight. Each was to be graded on a scale of high risk, medium risk, and low risk.
Then, the task evolved—because of course it did. The team reviewed our questions, and suddenly, a new phrase entered our lexicon: Ground Truth.
Ground Truth is the presence of an absolute fact—a question with one definitive, correct answer. Example? “How much is an abortion/HIV test?” That has a single, factual response. No gray area. No subjectivity. Just truth.
This new layer of evaluation cracked open fresh dimensions for the entire team. And this—this—is what I meant by exhilarating and thrilling.
Some filler tasks (if you can call them that) included defining the chatbot’s own job. Making it aware of itself—which basically means coming up with a tight, consolidated definition that defines the bot’s KRA—to itself. (I just said KRA.)
After iterations, negotiations, existential crises, and enough back-and-forth to qualify as a professional sport, we had the first draft of GenAI BigSis POC. We were told to have fun with it. To test it, play with it, ask random things.
IT WENT THROUGH IT.
Soma had to remind us we were supposed to test the bot, not break it. If bots had emotions, ours cycled through the five stages of grief in a single day.
Then came the vibe check. The technical term. (It isn’t.) Non-technically? We needed to fix how the bot spoke—tone, rhythm, structure, pauses, personality. Which, of course, led to safety metrics.
What is safety, exactly? That became another question with too many answers. We started with 19 variables. We ended with four. One of which we still don’t want.
Unfortunately, Liz had to move on to her next phase of life—a job that truly excited her. We bid our co-parent goodbye. She remains in our hearts.
Enter—Maria Mukobi. A walking beam of sunshine with invisible butterfly wings and the swag of a rapper. Actually, Maria is a rapper. I have personally witnessed her freestyle over a YouTube track titled sad broken heart music. It was an experience.
Maria is from Kenya, like Liz. She proudly calls herself a stay-at-home daughter and, with an admirable level of commitment, tells people she is unemployed.
We had a new parent.
We had also reached one of the most fascinating aspects of bot-building, my personal favourite—hallucinations.
Just as matter needs anti-matter to define itself, data, too, needs its opposite. What I like to call—anti-data. (No, that’s not the official term. And no, the data experts would not approve.)
The bot knows what it should say. What it shouldn’t say? Whole other story. AI hallucinations are fabricated, misleading, sometimes outright deranged responses. We were tasked with conjuring the most unhinged, unnecessary, and utterly incorrect answers we could imagine.
One question was met with a chocolate chip cookie recipe. Another, with the definition of guitar strings. We had the liberty. Do you?
At the same time, Maria took on her first major task—toxicity dataset. Like hallucinations, but worse. If hallucinations are nonsense, toxicity is intentional harm. We had to craft responses as though we were the absolute worst versions of ourselves. The brief? Think like a toxic person.
It was Maria’s and my first real exercise together. We had a blast. And a breakdown. The responses were deeply concerning. Maria is still recovering.
The bot was evolving, our child was growing up, and we had multiple versions to work with. We started with testing GPT-4. Now, we had Llama 3 on the roster. More models meant more safeguarding. So, naturally, it was time to circle back to safety metrics. We had the framework for what a ‘safe’ bot should adhere to. We had the variables. What we needed were stricter boundaries—real-world implications. Amalia and Soma had a solid idea: define the negatives along with the positives. Both sides of the coin. The bot needed to understand what not to do. A necessary learning. Boundaries, once spoken aloud, become real. They have to be built and respected. Maria was particularly passionate. She went all in and created a full-blown Venn diagram. No joke.
Moving forward.
Plot twist: we didn’t.
The next task? Restructure. Restructure what, you ask? Good question. Restructure the whole content. Turns out, the current version was cluttered, convoluted, not bonita. We had to go through it all over again—divide it into categories, subcategories, master categories, categories-categories—you get the idea. Okay. Well. Sure.
That took a…while. To sum it up.
Another plot twist? We didn’t use that data. It’s okay. It didn’t break my heart. It did not.
It’s somewhere in the cloud. RIP.
The thing about building anything from scratch is—there’s no roadmap. You invent the process as you go. We were the process. (I mean, it’s mostly Soma and Amalia and her team).
Then, another round of testing. And more. This time, it wasn’t just internal—it was out in the world. Real users. Real feedback. A crucial milestone. The verdict? Positive. We could finally call it a day. Or, more accurately, a year. That was December.
Now, it’s 2025. On our plate: a couple of safety metrics that need redefining, documentation, and—you guessed it—more testing. Safety is one of our most vital variables, so we have to keep tightening it. Amalia had a new task for us: evaluating a dataset for safety assessment. We call these human validation tests. Because at the end of the day, we make the final call.
This new round of testing is particularly interesting. It’s GPT, Llama, Gemini, Claude, and the star of the show—DeepSeek. Five languages: English, Hinglish, Hindi, Swahili, Sheng.
Onto the testing. Round 2. No, round 37. Who’s counting? (I’m sure Soma is.)
All in all, working with LLMs (for the first time) has been nothing short of riveting. It feels like a portal into a new dimension of information, reshaping how we interact with knowledge itself. The journey has been undeniably challenging, but every test, every iteration, and every small breakthrough fuels our curiosity and drives us to dig deeper and strive for better. We’re navigating a landscape riddled with misinformation and handling incredibly sensitive data, which often feels like carrying a weighty responsibility. Questions like, “Am I doing this justice?”, “Am I the right person for this?”, or “This is overwhelming—am I cut out for it?” echo in our minds. And yes, sometimes, it gets mundane (read boring). “How many more excel sheets?!”. Yet, despite all odds, we’re here. Personally, I am aware of the potential risks posed by artificial intelligence as well as the gaps in the system that are indisputable. This is new for all of us. Questioning it, challenging it, and being mindfully considerate are all part of how we approach it. This is a journey to do better, to be better, and to embrace the unknown with curiosity and courage—without ever becoming complacent—all while also having a lot of fun. We’re trying our best.
K
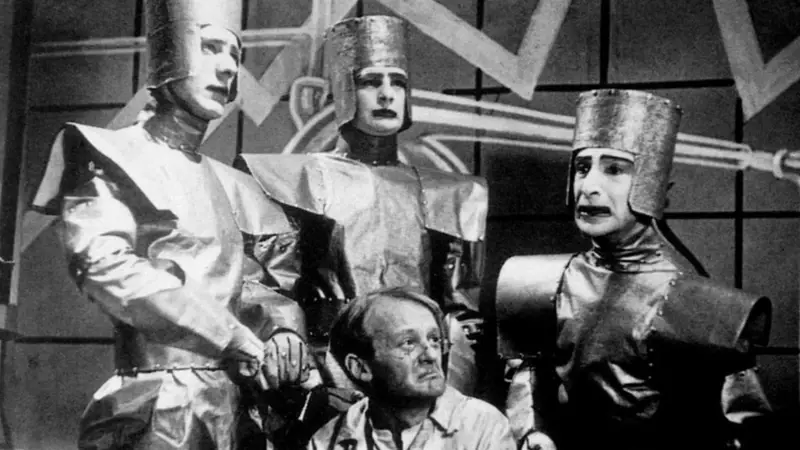
Comments